Hi, I’m Yosefine, a Human Resources Intern at Bukit Vista. Today, I bring you an article about the intersection of data science and the ever-evolving landscape of the hospitality industry. On the next paragraph, you will join me on a captivating journey led by Mr. Slawek Smyl, a leading figure in data science. This article offers an in-depth exploration of machine learning methods, loss functions, the art of continuous learning in data models, hyperparameter optimization, and the strategies needed to face unexpected challenges. As you dive into the effort to explain the model, you’ll see why this article is an invaluable resource for those looking to uncover the ins and outs of data science in the context of our dynamic hospitality world.
Nestled in the heart of Bali’s vibrant hospitality industry, Bukit Vista isn’t just a player; it’s a visionary force. Beyond offering guests a comfortable stay, we aspires to become an integral part of the professional network for hospitality innovation. Our mission is clear – to inspire delight at every turn, in every corner of the world. This isn’t just a mission statement; it’s a way of life, etched into our Core Values, and shared passionately with our community. In return, we have nurtured a self-driven community, all united by one purpose – to inspire delight.
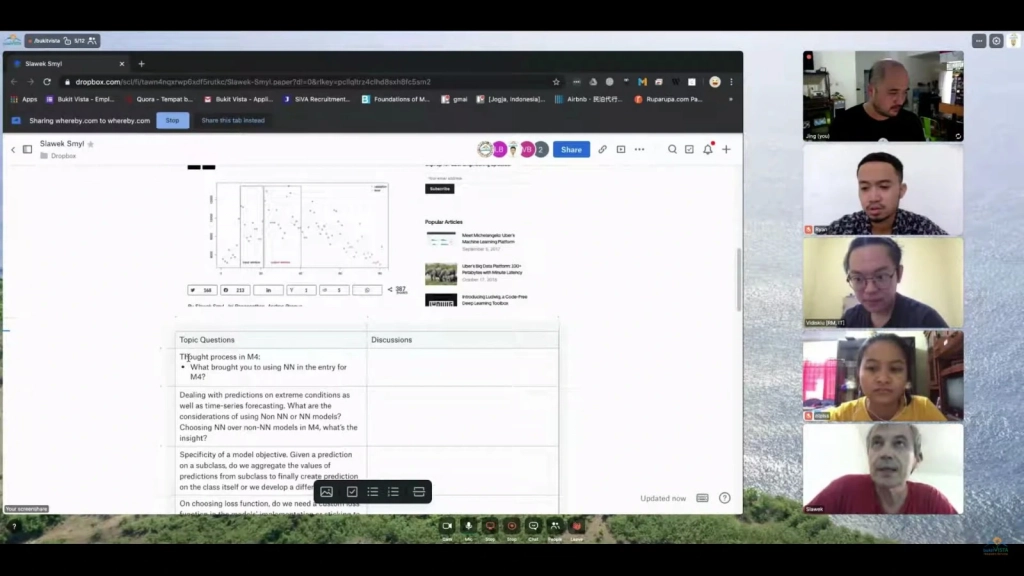
Our journey into the realm of data science is led by Vidiskiu, a guiding light in this exploration. As we step into this world, we meet Mr. Slawek Smyl, Uber Data Science renowned for his pioneering research in machine learning methods. Vidi dives into the intriguing decision-making process behind Slawek’s choice to blend non-neural network methods with neural networks during the M Four competition.
Slawek takes us back to the roots of his decision – a moment when traditional methods fell short in forecasting Azure’s growth rate. This led him to discover the revolutionary program called Stan and, subsequently, dynamic computational graph neural networks. These discoveries empowered him to craft his own models, setting the stage for success even before the M Four competition commenced.
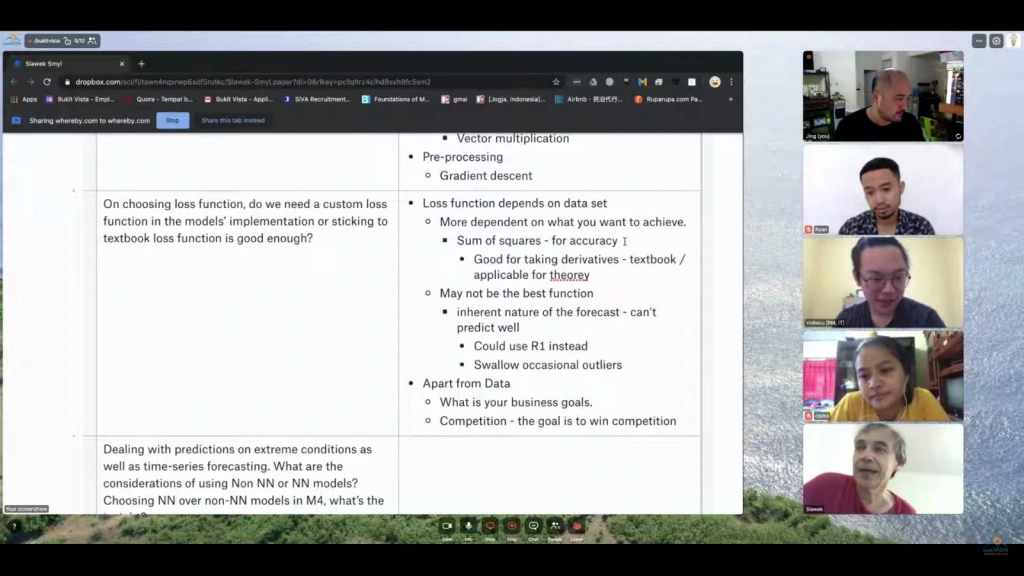
The conversation then shifts to a critical aspect of data science – loss functions. Slawek unveils the intricate process of selecting the right loss function, emphasizing that it hinges on the desired outcome and its alignment with business objectives or competition criteria. He sheds light on the adaptability of customized loss functions, tailored to specific needs, such as giving priority to outliers or addressing specific error types.
In the dynamic world of data models, continuous learning is paramount. Slawek offers his insights on striking the delicate balance between starting from scratch and fine-tuning existing models with new data. It’s a nuanced decision that depends on the business cycle and data availability. As he explains, it’s about integrating new data while retaining the valuable features from previous production models.
The discussion takes a fascinating turn as we explore the optimization of hyperparameters using genetic algorithms and neural networks. Slawek introduces us to the concept of using genetic algorithms for optimization, although he acknowledges the time constraints of this approach. Bayesian optimization emerges as a practical alternative.
As we navigate the complexities of data modeling, the topic shifts to preparing for unprecedented situations like the COVID-19 pandemic. Slawek suggests scaling down existing models and monitoring external indicators, such as economic indexes or hospital bed occupancy rates. However, he also acknowledges the challenges of modeling predictions when past patterns no longer apply. Incorporating external data sources becomes essential, provided sufficient historical data is available.
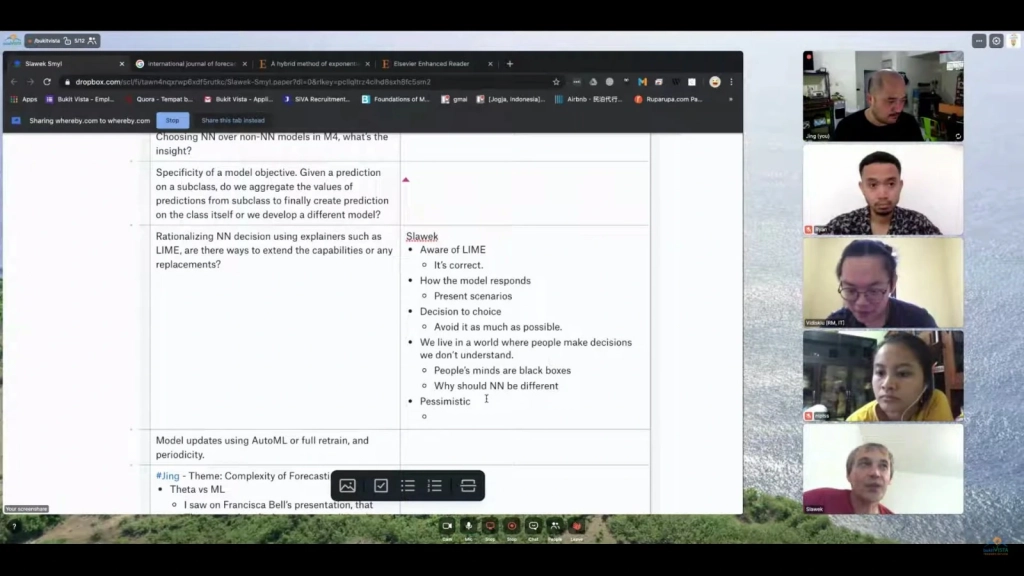
In the ever-evolving landscape of data science, explaining model results, particularly in black box neural network models, poses significant challenges. Slawek introduces Lime, a tool commonly used in Indonesia to explain model decisions. However, he highlights the limitations of explainability methods, emphasizing that business decision-making encompasses multifaceted factors beyond what these methods can unravel.
In this journey through the world of data science, guided by Mr. Slawek Smyl, we’ve uncovered the intricacies of combining machine learning methods, crafting customized loss functions, and navigating the ever-changing landscape of data models. As we continue to explore the uncharted territories of predictive analytics, one thing is certain – the future belongs to those who can decipher its patterns, and with luminaries like Mr. Slawek Smyl leading the way, we’re poised to unlock its many secrets.